Have you ever wondered how businesses make sense of the overwhelming amounts of data they encounter daily? Have you heard about the term "unstructured data" and how artificial intelligence (AI) is used to interpret it? If these questions intrigue you, then you're in the right place!
Unstructured Data: A Quick Overview
Data. It's the lifeblood of today's digital age. There's structured data, the neat and orderly stuff that fits into spreadsheets with rows and columns.
Then there's unstructured data, the wild child of the data family. Unstructured data comes in many forms: emails, social media posts, customer reviews, videos, audio files, and more.
This data doesn't fit neatly into traditional databases, but it holds valuable insights businesses can leverage. And with 80% of the world's data being unstructured, it's a goldmine waiting to be unearthed!
Artificial Intelligence: Breaking Down the Basics
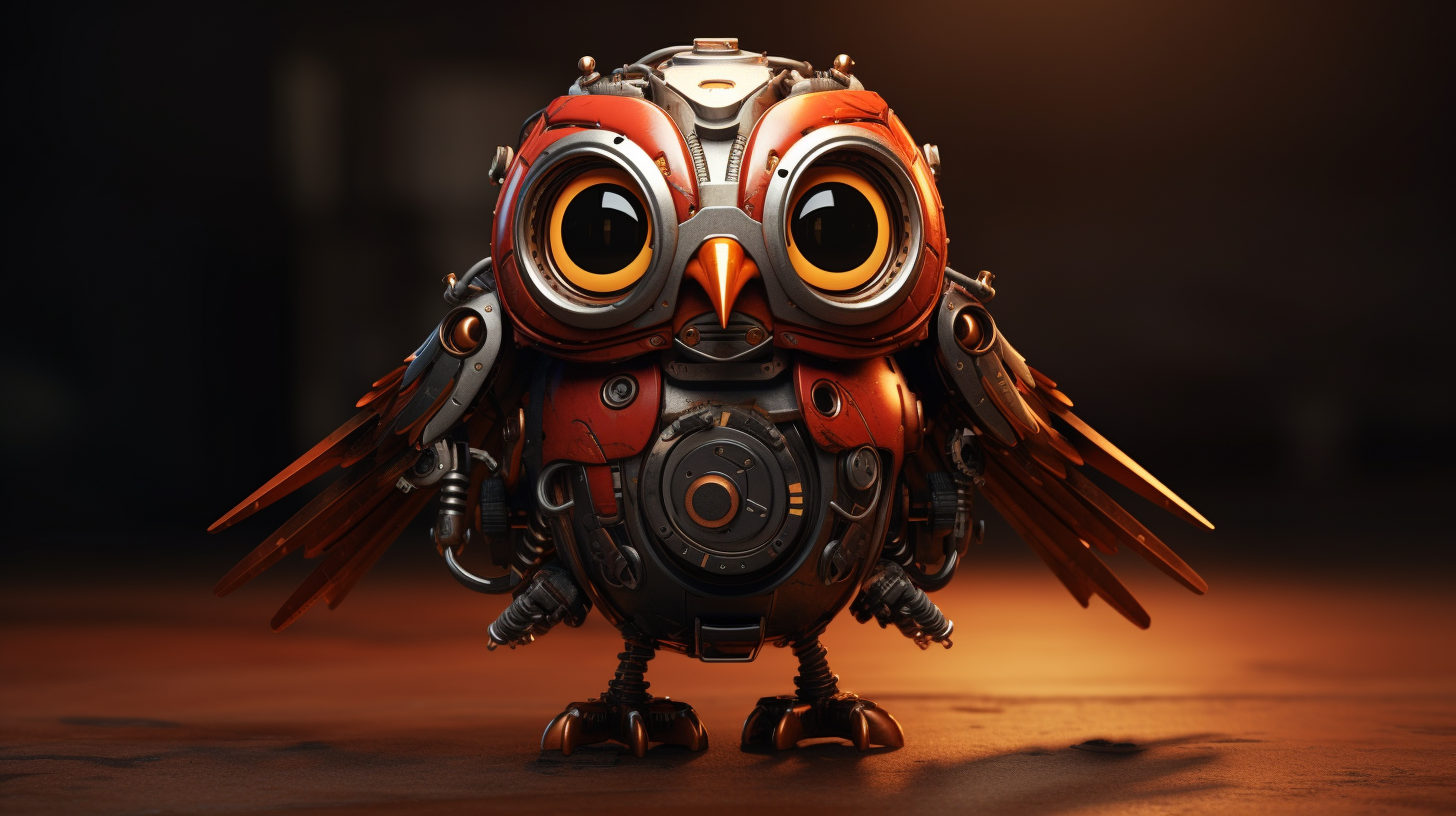
Artificial Intelligence, or AI, might sound like it belongs in a galaxy far, far away, but it's much closer to home than you might think.
At its core, AI is about creating machines that mimic human intelligence. These machines can analyze data, recognize patterns, make decisions, and even learn from experience. But here's where it gets really interesting.
The way AI learns is vastly different and more powerful than human learning, especially when lots of data are involved. As humans, our brains are extraordinary, but even they have limits.
Take our short-term memory, for instance. Ever heard of the magic number seven? Psychology tells us that we can hold about seven (plus or minus two) items in our short-term memory. That's not a lot, especially in today's data-driven world!
Now, consider AI and machine learning. These systems can handle mind-boggling amounts of data, detecting patterns and drawing conclusions on a scale that's simply beyond human capability. Unlike humans, AI doesn't get overwhelmed by large data sets. Instead, it thrives on them.
Let's illustrate this with an example. Imagine you're running a global e-commerce business with millions of customers.
Each customer interaction, product review, transaction, and click on your website is a piece of data. Now, a human brain would struggle to make sense of all this information. But AI? It's a walk in the park.
AI can analyze this massive data, identify purchasing patterns, predict future trends, and personalize the customer experience. It can even learn from past data to improve future predictions, constantly refining and updating its algorithms.
The Connection Between AI and Unstructured Data
With a clearer understanding of AI and unstructured data under our belts, it's time to examine how these two fields interact. The combination of AI and unstructured data is like pairing a master detective with an untapped goldmine of information.
AI is the modern-day Sherlock Holmes, using powerful tools like Natural Language Processing (NLP) and machine learning to make sense of the vast uncharted territories of unstructured data.
How AI Interacts with Unstructured Data
AI uses machine learning to sift through mountains of unstructured data, spotting patterns and pulling out insights that would otherwise go unnoticed.
For example, AI could analyze hundreds of thousands of social media posts to understand trending customer sentiment towards a brand, or scan through stacks of documents to extract key information.
But where AI really shines is in handling the complexities of unstructured data, such as text, images, or audio from online meetings. And this is where tools like NLP and machine learning become invaluable.
AI Technologies Leveraged for Unstructured Data
Natural Language Processing (NLP)
NLP is a branch of AI that allows machines to understand, interpret, and generate human language. It's like teaching a machine to read, comprehend, and converse.
It is NLP that powers our digital assistants, translates text into different languages, and enables machines to understand the nuances of our language, like context, sentiment, and intent.
In a business setting, NLP can transform unstructured data from online meetings into structured insights.
For example, NLP can transcribe spoken language into written text, identify key discussion points, assign tasks mentioned during the meeting, and even gauge the sentiment of the conversation.
Machine Learning (ML)
Consider the case of a customer service team that holds weekly online meetings to discuss customer issues and feedback.
A machine learning model could be trained on the transcripts of these meetings, along with the corresponding customer satisfaction scores and feedback from the same time frame.
Over time, this model could learn to identify patterns or recurring issues discussed in these meetings that lead to lower customer satisfaction scores.
For instance, it might find that whenever there are discussions around delays in shipping or technical glitches in the website, customer satisfaction tends to dip.
Once the model has been adequately trained, it can then analyze the transcripts of current meetings in real-time and flag if similar issues are being discussed.
This could serve as an early warning system, allowing the company to proactively address these issues before they significantly impact customer satisfaction.
The Impact of AI and Unstructured Data in Business
Businesses that harness the power of AI and unstructured data gain a competitive edge. They can uncover hidden insights, make better decisions, and offer personalized experiences to their customers.
Making Sense of Unstructured Data
Consider unstructured data as pieces of a jigsaw puzzle. Each piece, on its own, might seem insignificant. For instance, imagine a customer leaves a review on your e-commerce platform about a product they purchased. Alone, it's just a single opinion. It doesn't give you a broad overview.
However, let's take AI into the equation. The AI system gathers thousands of these individual pieces of feedback. It doesn't just look at reviews on your platform, but also scours social media comments, blog post discussions, email feedback, and even transcribes and analyzes voice comments from customer service calls.
As these puzzle pieces start fitting together, a comprehensive picture emerges. You begin to see patterns. Perhaps a particular product feature is consistently praised. Maybe there's a recurrent issue with shipping times. It could even be a subtle shift in customer preferences, such as a growing demand for eco-friendly packaging.
AI-driven Business Decisions
Armed with these insights, businesses can make data-driven decisions. Whether it's identifying potential risks, spotting opportunities, or understanding customer behavior, AI provides the tools to make informed choices.
AI can also help businesses manage risk and ensure compliance. It can monitor data for unusual activity, flagging potential issues before they become problems.
Case Studies of AI and Unstructured Data in Action
To make this more concrete, let's look at some real-world examples.
Case Study 1: Online Fashion Retailer's AI Revolution
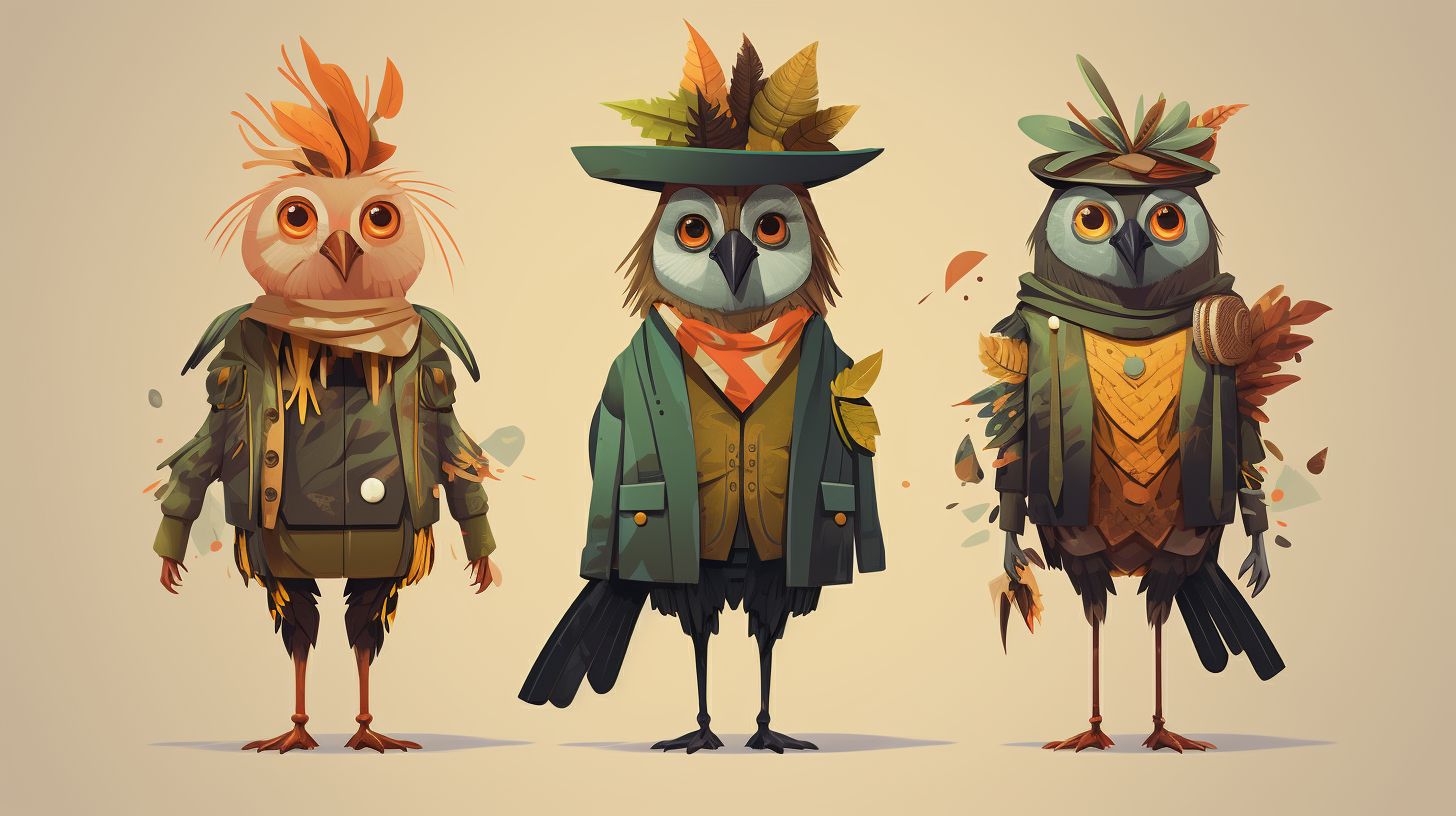
Once upon a time, a major online fashion retailer, let's call them "TrendSetter," was facing a particular challenge. With a customer base in the millions, TrendSetter was flooded with reviews and social media posts. It was like trying to listen to a thousand voices at once - they knew valuable feedback was there, but making sense of it all was a different story.
TrendSetter decided to leverage AI to help them tune into this cacophony of customer voices. They built a system that used Natural Language Processing (NLP) to dissect this barrage of unstructured data, extracting customer sentiment and preferences.
One particular insight was a game-changer. The AI system detected an increasing sentiment towards sustainable fashion in customer reviews and social media posts. Customers were increasingly concerned about the environmental impact of their purchases and wanted more eco-friendly options.
Acting on this insight, TrendSetter launched a new "Eco-Friendly" line made from sustainable materials. They highlighted the sustainability efforts in their marketing campaigns, directly addressing the concerns voiced by their customers. This move was a hit - customers felt heard, and TrendSetter saw a significant uptick in customer engagement and sales, proving that the investment in AI paid off handsomely.
Case Study 2: Financial Institution Leverages AI to Streamline Loan Approvals
Aleading bank was wrestling with the time-consuming loan approval process. A loan application at the bank was like a mini-biography of a person, filled with financial details, personal history, and more.
SureBank decided to employ AI to streamline their loan approval process. They developed a system that utilized machine learning to scrutinize loan applications.
During the initial phase of AI implementation, the system flagged a recurring pattern in high-risk applications: applicants with a history of multiple, simultaneous short-term loans often defaulted. Armed with this insight, the bank refined their risk assessment parameters, giving more weight to this particular risk factor.
The result was a substantial reduction in bad loans, a faster loan approval process, and a decrease in error rates. The AI system was able to identify risk patterns humans could have easily missed, making SureBank's loan process smarter and more efficient.
Case Study 3: AI-Driven Healthcare Revolution
Keeping up with the latest medical research is akin to drinking from a firehose. A leading healthcare provider found themselves grappling with this challenge. They had access to vast amounts of patient records and new research papers but needed a way to connect the dots efficiently.
They employed AI to tackle this. They used NLP to analyze patient records and machine learning to scan through research papers.
One specific application had a transformative impact. The AI system flagged a new research paper about the effectiveness of a specific drug in managing a rare chronic condition. The provider had several patients suffering from this condition and were struggling with its management.
Armed with this new research insight, its doctors were able to prescribe the righ tdurg leading to better management of the condition and improved patient outcomes. This example demonstrated the power of AI in translating unstructured data into tangible actions, resulting in enhanced healthcare delivery.
Case Study 4: Transforming Online Meetings with AI and Unstructured Data
The shift to remote work during the recent years brought with it an explosion of virtual meetings. Suddenly, businesses found themselves sitting on a treasure trove of unstructured data: meeting recordings. But how to mine this gold? Enter AI.
A leading global technology company saw an opportunity here. They had always prided themselves on fostering innovation through collaboration and wanted to find a way to capture the wealth of knowledge and ideas exchanged in these online meetings. However, manually going through hours of video recordings was unfeasible. They needed a more efficient approach.
The company decided to leverage AI, specifically machine learning and NLP, to handle this unstructured data. Their goal was twofold:
- To extract key insights and decisions from meetings to ensure nothing valuable was lost in the information overload.
- To analyze the communication patterns and feedback sentiments for continual improvement in their teams' collaboration and interaction.
The company developed an AI-driven tool that transcribed meeting recordings and analyzed the content. NLP was used to understand the context and extract essential points, decisions made, tasks assigned, and even detect the sentiments expressed by the participants.
This implementation was a game-changer. Key meeting insights could be quickly reviewed and shared, ensuring everyone stayed in the loop and important tasks or decisions didn't fall through the cracks. It was especially helpful for team members in different time zones or those who couldn't attend the meeting.
Moreover, by analyzing meeting dynamics and sentiments, the company could make data-driven decisions to improve their meeting effectiveness. For instance, if the AI detected negative sentiments during a particular project discussion, leaders could promptly address the concerns, preventing potential issues from escalating.
In terms of data privacy, the company implemented strict measures to anonymize data and ensure only high-level insights were shared, respecting individual privacy.
This case study beautifully showcases the power of AI in handling unstructured data, even in areas we might not typically think about, like online meetings. As we move forward, the use cases of AI in managing unstructured data will only continue to grow, offering exciting opportunities for businesses to drive innovation and efficiency.
Challenges and Solutions in Leveraging AI for Unstructured Data
While AI provides a powerful lens to examine unstructured data, like all powerful tools, it presents unique challenges. However, with thoughtful planning and responsible use, these hurdles can be overcome.
Challenge 1: Data Privacy
Data privacy is akin to a sacred pact between businesses and customers. With AI's ability to delve deep into unstructured data for insights, respecting this pact can seem like a tightrope walk. How can businesses ensure that they respect customer privacy while extracting valuable insights from data?
Solution 1: Anonymization and Encryption
Businesses can turn to techniques like anonymization and encryption. Anonymizing data involves removing any personally identifiable information (PII) from the data set, making it impossible to trace the data back to an individual. On the other hand, encryption translates data into another form or code, so only people with access to a secret key can read it.
These techniques ensure that the essence of the data remains intact for analysis while protecting individual privacy. Thus, businesses can explore unstructured data landscapes without infringing on privacy rights.
Challenge 2: Data Quality
The age-old adage of 'garbage in, garbage out' holds particularly true for AI. The quality of insights is only as good as the input data. With unstructured data often being messy and inconsistent, how can businesses ensure the insights derived are not skewed or incorrect?
Solution 2: Data Validation Techniques
Data validation techniques come to the rescue here. These include methods to clean, standardize, and validate data before it is analyzed. For instance, eliminating duplicate entries, correcting inconsistent formatting, or filling missing values. By ensuring that the input data is as accurate and reliable as possible, businesses can trust the insights generated by AI.
Challenge 3: Scalability
As businesses grow, so does their data. Handling a few gigabytes of data might be manageable, but what about when it escalates to terabytes or even petabytes? How can businesses scale their AI solutions to keep up with this mountain of data?
Solution 3: Vector Embeddings
To tackle this challenge, there's a smart technique called vector embeddings. Now, you might think this sounds too technical, but let's break it down with a simple analogy.
Let's imagine each piece of data is like a unique book in a gigantic library. Now, if you had to search for books on similar topics, how would you do it? You could read every book, but that would take an eternity! Instead, you'd probably categorize books based on their topics, right? This way, similar books are grouped together, making your search much easier and faster.
Vector embeddings do the same for your data. They group similar data points together in a way that our AI system understands. So, instead of going through every data point separately, your AI system can now quickly look at these groups for faster, more efficient analysis.
Conclusion
The role of AI in business isn't just a fleeting trend - it's becoming the standard. The ability to sift through mountains of unstructured data and extract valuable insights is becoming a fundamental aspect of business operations, from customer sentiment analysis to risk assessment, product development, and beyond.
By understanding the concepts behind AI and unstructured data, businesses can navigate this new landscape more effectively. Through real-world case studies, we've seen the transformative potential of AI, its ability to uncover insights that can shape business strategies, and the specific challenges and solutions associated with it.
Of course, applying AI to unstructured data isn't without its difficulties, but as we've explored, solutions like data anonymization, encryption, data validation, and vector embeddings offer effective ways to overcome these challenges.
Wudpecker stands the forefront of this AI-driven revolution. As an AI meeting tool, we empower professionals to find critical answers from their online meetings. By applying the principles and techniques discussed in this blog, we're able to delve into vast oceans of unstructured data, extract key insights, and deliver them right at your fingertips.
